日本ソフトウェア科学会
機械学習工学研究会
国際シンポジウム 2019

2019/10/18(金) 13:30〜17:45
(プレイベント 9:30~12:00 交流会 18:00〜20:00)
一橋大学 一橋講堂 (東京都千代田区)
お知らせ
本イベントは終了いたしました。
ご支援・ご参加いただいた皆様ありがとうございました。
質問・議論テーマ受付先: https://app.sli.do/event/dumhcj5o/live/questions
( https://www.sli.do/ からイベントコード #MLSE19 入力でも可)
当日受付ではconnpassで発行できる受付票をご提示ください。
10/15: 台風の影響により一部講演者の来日に未だ調整が付かず、
プログラムを大きく変更させていただきました。
今後および当日の状況により多少の変更が発生する可能性もあります。
どうぞご了承下さい。
→ 参加登録はこちらから
近年の機械学習、特に深層学習(ディープラーニング)の技術発展に伴って、機械学習を利用するシステムは急速に社会に浸透しつつあります。しかし、機械学習を利用するシステムの開発や運用、保守においては、従来のソフトウェアシステムとは異なる課題が存在し、新しい原則、アプローチ、技術が求められています。この潮流は日本国内に限らず、海外においても目立ってきており、産官学において様々な研究や実践の試みがなされています。
設立2年目を迎えた日本ソフトウェア科学会 機械学習工学研究会(MLSE)においては、ますます重要となる機械学習工学に関し、情報交換と議論を行うシンポジウムを開催いたします。本シンポジウムは、海外のトップ研究者が日本に集う「湘南会議」というイベントに連動して開催しており、海外からの第一線研究者6名の講演があります(同時通訳あり)。他にも海外からの研究者多数が参加予定です。
本シンポジウムにおいては特に、自動運転などの応用を見据えて安全性・信頼性に関する
研究に取り組んでいる第一線の研究者による招待講演複数が目玉となっています。
ただしそれらに話題を限ることなく、広い観点からの討論も行います。
参加登録
本イベントは終了いたしました。
(参加登録サイトはこちら)
プログラム
10/15: 台風の影響を受けて大きく変更いたしました。
今後および当日の状況により多少の変更が発生する可能性もあります。
09:30~12:00 午前の部 (Seshia氏は英語で実施,他は英語スライド・日本語で実施)
9:30~9:35 オープニング
9:35~10:10 キックオフ招待講演 Sanjit Seshia 氏 (リモート):
Towards Verified Artificial Intelligence
10:10~10:40 イントロダクション 石川 冬樹 :
MLSE研究会および関連する活動・動向の報告
10:40~10:50 休憩
10:50~11:50 プレ講演 蓮尾 一郎 氏 :
Safety under Statistical and Environmental Uncertainties:
Challenges in Cyber-Physical Systems with Machine-Learning Components
11:50~12:00 質問
13:30~18:05 午後の部 (英語で実施・同時通訳あり)
13:30-13:35 イントロダクション
13:35-14:45 招待講演(1) 榊原 彰 氏 :
Engineer's Responsibility in Machine Learning Era
14:45-15:05 休憩
15:05-16:15 招待講演(2) Foutse Khomh 氏 :
Towards Debugging and Testing Deep Learning Systems
16:15-16:25 休憩
16:25-17:05 パネル討論に向けた話題提供
Lei Ma 氏, Amel Bennaceur 氏, Ruediger Ehler 氏
17:05-18:05 パネル討論
18:15~20:15 交流会(展示あり)
登壇者
Sanjit Seshia
カリフォルニア大学バークレー校(アメリカ)
Towards Verified Artificial Intelligence
(台風のためリモート実施に変更)
蓮尾 一郎
国立情報学研究所
Safety under Statistical and Environmental Uncertainties: Challenges in Cyber-Physical Systems with Machine-Learning Components
Krzysztof Czarnecki
ウォータールー大学(カナダ)
Uncertainty-Centric Safety Assurance of ML-Based Perception for Automated Driving
(台風のためキャンセル)
基調講演者
Foutse Khomh
モントリオール大学(カナダ)
Towards Debugging and Testing Deep Learning Systems
The increasing inclusion of Deep Learning (DL) models in safety-critical systems such as autonomous vehicles have led to the development of multiple model-based DL testing techniques. One key limitation of these approaches is the lack of diversity in their generated test cases, which often results in poor performance. Also, they assume that training programs are adequate and bug-free. An assumption that is not always true since DL programs like any software program can contain bugs. In this talk, I will introduce the audience to a TensorFlow-based library that we have developed to help DL engineers detect issues in their training programs. I will also present DeepEvolution, a novel search-based approach for testing DL models that relies on meta-heuristics to ensure a maximum diversity in generated test cases.
榊原 彰
日本マイクロソフト
Engineer's Responsibility in Machine Learning Era
To appear
Lionel Briand
ルクセンブルク大学(ルクセンブルク)
オタワ大学(カナダ)
Testing Autonomous Systems: Challenges and Strategies
(台風のためキャンセル)
Increasingly, autonomous systems’ behavior is driven my machine learning, and in particular by (deep) neural networks. One crucial question then is how to effectively test such systems given that they don’t have (complete) specifications or even source code corresponding to some of their critical behavior. Further, adding even more complexity to an already difficult problem, many of these autonomous systems are usually integrated into larger systems, thus possibly leading to undesirable feature interactions. This talk will report on recent work done to address these intricate problems and will attempt to better define the challenges ahead. Examples from the automotive domain will be used to illustrate the main points.
交流会
18:00~20:00
展示会を含めた交流会を行います。
ゴールドスポンサー
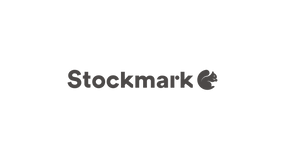


株式会社日立製作所
特別スポンサー
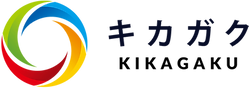
メディアスポンサー

協賛・後援
電子情報通信学会 知能ソフトウェア工学研究会 (SIG-KBSE)
電子情報通信学会 情報論的学習理論と機械学習研究会(IBIS-ML)
日本ディープラーニング協会(JDLA)
情報処理学会 ソフトウェア工学研究会(SIG-SE)
一般社団法人JASPAR
NPO法人ソフトウェアテスト技術振興会(ASTER)
日本ソフトウェア科学会 ソフトウェア工学の基礎研究会(FOSE)
人工知能学会(JSAI)

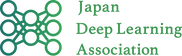
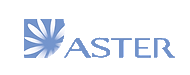
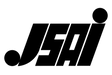
スポンサー・協賛
アクセス
一橋大学 一橋講堂
東京都千代田区一ツ橋 2-1-2 学術総合センター内
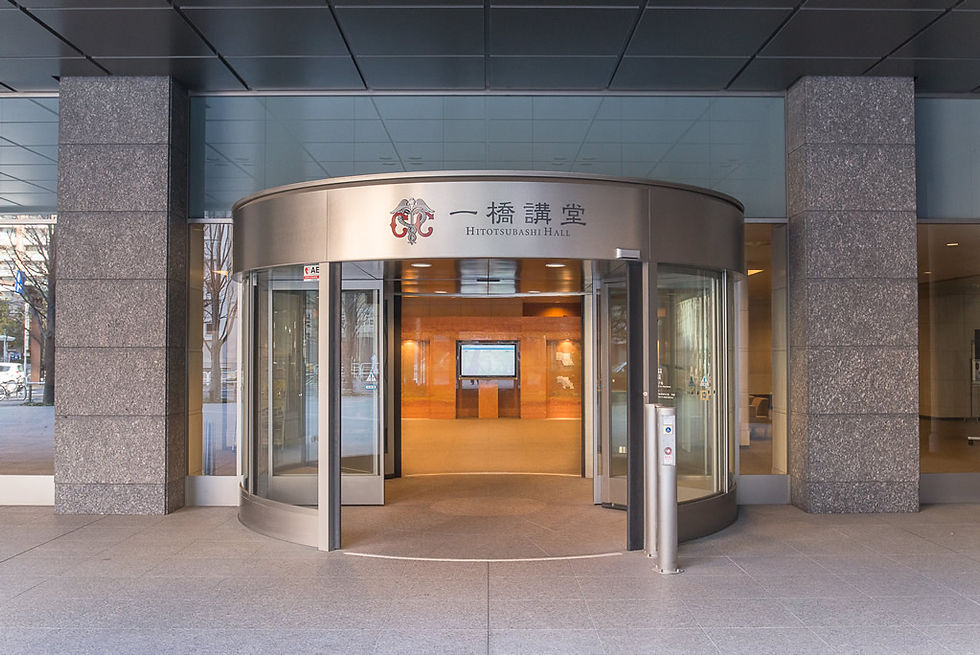
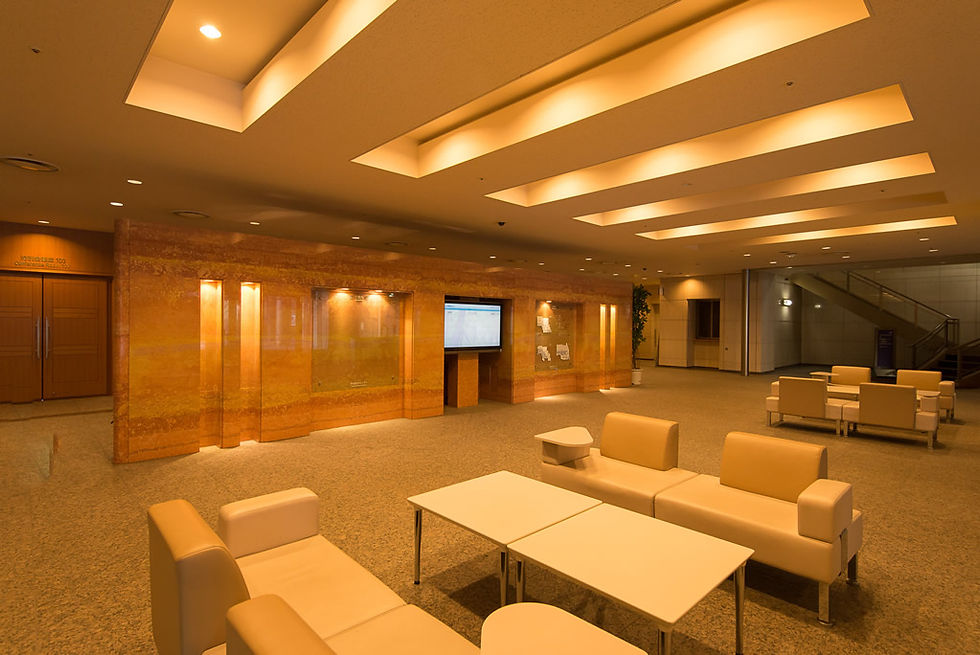
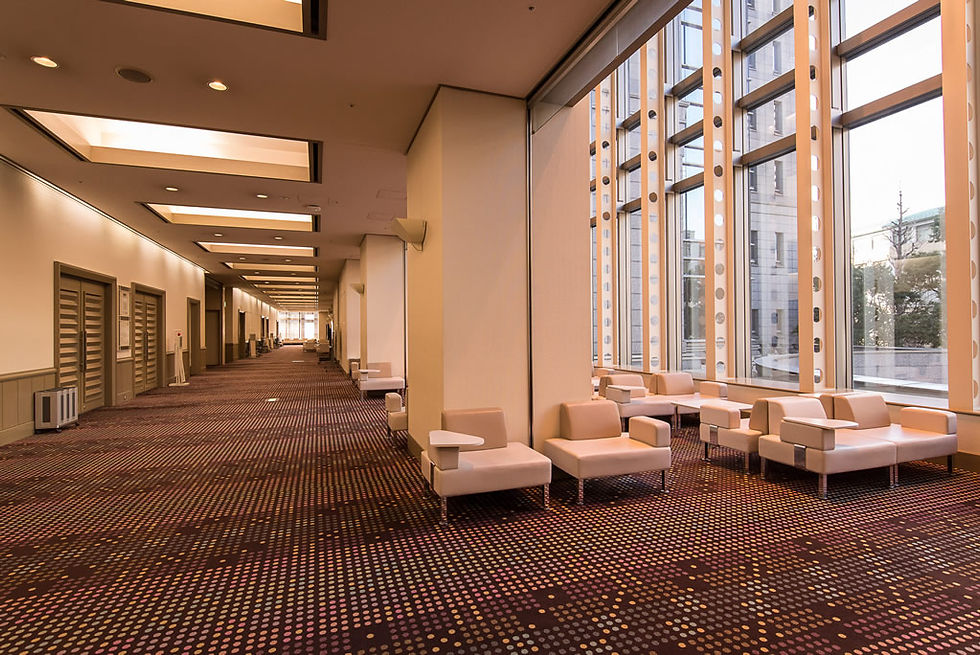
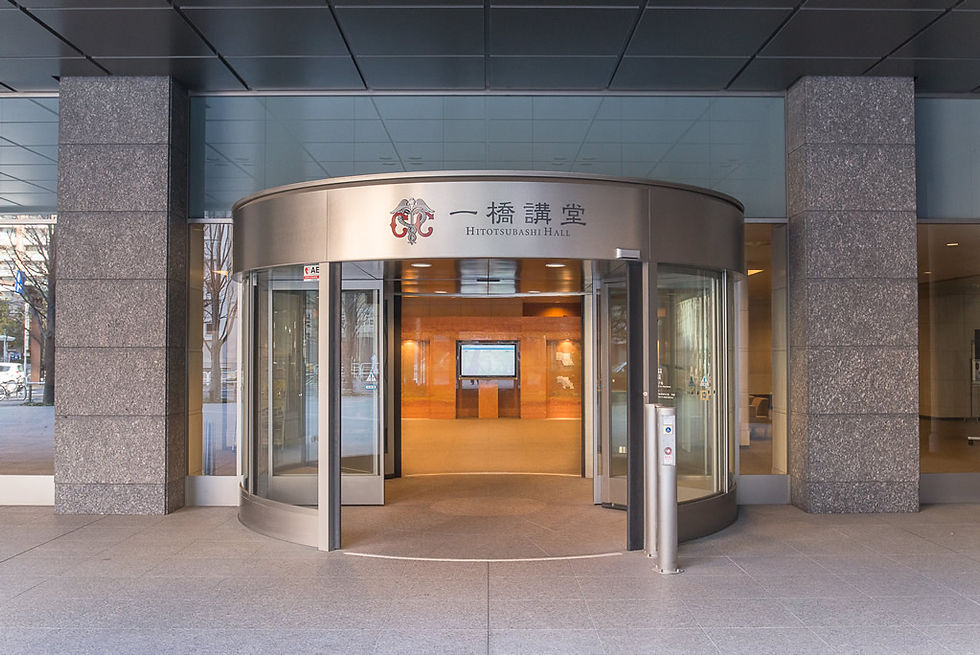
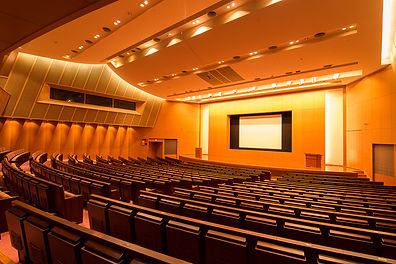