MLSE International
Symposium 2019

2019/10/18 (Fri) 13:30〜17:45
(Pre-event 9:30 - 12:00 Social dinner 18:00 - 20:00)
Hitotsubashi Hall (2-1-2 Hitotsubashi, Chiyoda-ku, Tokyo)
News
This event has successfully ended. Thank you for your support and participation.
Questions and Discussion Topics are collected at :
https://app.sli.do/event/dumhcj5o/live/questions
Oct 15 : Due to the typhoon, trips of some speakers were cancelled.
We have largely changed the program and may have slight changes.
Attendees of Shonan Meeting 156 (SEMLS) do NOT need to make the registration.
Applications of machine learning (ML)-based systems have been actively investigated given the recent advance of ML techniques, especially deep learning. However, there are unique difficulties in development, operation, and maintenance of ML-based systems. There is thus increasing demand for advanced principles, approaches, and techniques beyond those in traditional software engineering. This trend is triggering various efforts in research and practice in Japan and in the world.
This symposium is organized by SIG-MLSE, Special Interest Group on Machine Learning System Engineering in JSSST. The objective is to promote exchange and discussion on the MLSE area. Linked with Shonan Meeting, this symposium will welcome researchers from all over the world, to be connected with the academia and industry in Japan.
Registration
This event has ended.
(registration site : https://mlxse.connpass.com/event/147250/)
Attendees of Shonan Meeting 156 (SEMLS) do NOT need to make the registration.
Program
We have largely changed the program and may further have slight changes.
9:30~12:00 Morning (English slides, Talk in Japanese, except for Prof. Seshia)
9:30~9:35 Opening
9:35~10:10 Kick-off Invited Talk Sanjit Seshia (remote) :
Towards Verified Artificial Intelligence
10:10~10:40 Introduction Fuyuki Ishikawa :
Report of MLSE and relevant activities
10:40~10:50 Break
10:50~11:50 Preliminary Talk Ichiro Hasuo :
Safety under Statistical and Environmental Uncertainties:
Challenges in Cyber-Physical Systems with Machine-Learning Components
11:50~12:00 Questions and Discussions
13:30~18:05 Afternoon
13:30-13:35 Introduction
13:35-14:45 Invited Talk (1) Akira Sakakibara :
Engineer's Responsibility in Machine Learning Era
14:45-15:05 Break
15:05-16:15 Invited Talk (2) Foutse Khomh :
Towards Debugging and Testing Deep Learning Systems
16:15-16:25 Break
16:25-17:05 Position Talks Lei Ma, Amel Bennaceur
17:05-18:05 Panel Discussion
18:15~20:15 Social Dinner (including Exhibitions)
Speakers
Sanjit Seshia
University of California, Berkeley, US
Towards Verified Artificial Intelligence
(Changed to Remote Presentation due to Typhoon)
Ichiro Hasuo
National Institute of Informatics, Japan
Safety under Statistical and Environmental Uncertainties: Challenges in Cyber-Physical Systems with Machine-Learning Components
Lei Ma
Kyushu University, Japan
Towards Testing and Analysis of Deep Learning Systems
Krzysztof Czarnecki
Waterloo University, Canada
Uncertainty-Centric Safety Assurance of ML-Based Perception for Automated Driving
(Cancelled due to Typhoon)
Keynote Speakers
Foutse Khomh
Univerisity of Montreal, Canada
Towards Debugging and Testing Deep Learning Systems
The increasing inclusion of Deep Learning (DL) models in safety-critical systems such as autonomous vehicles have led to the development of multiple model-based DL testing techniques. One key limitation of these approaches is the lack of diversity in their generated test cases, which often results in poor performance. Also, they assume that training programs are adequate and bug-free. An assumption that is not always true since DL programs like any software program can contain bugs. In this talk, I will introduce the audience to a TensorFlow-based library that we have developed to help DL engineers detect issues in their training programs. I will also present DeepEvolution, a novel search-based approach for testing DL models that relies on meta-heuristics to ensure a maximum diversity in generated test cases.
Akira Sakakibara
Microsoft Japan
Engineer's Responsibility in Machine Learning Era
To appear
Lionel Briand
Testing Autonomous Systems:
Challenges and Strategies
(Cancelled due to Typhoon)
Increasingly, autonomous systems' behavior is driven my machine learning, and in particular by (deep) neural networks. One crucial question then is how to effectively test such systems given that they don’t have (complete) specifications or even source code corresponding to some of their critical behavior. Further, adding even more complexity to an already difficult problem, many of these autonomous systems are usually integrated into larger systems, thus possibly leading to undesirable feature interactions. This talk will report on recent work done to address these intricate problems and will attempt to better define the challenges ahead. Examples from the automotive domain will be used to illustrate the main points.
Social Dinner
18:00~20:00
Includes demonstration by sponsor companies
Gold Sponsors
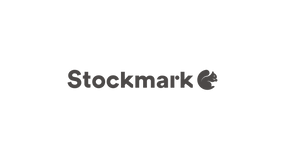


株式会社日立製作所
Media Sponsors
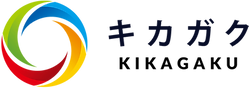
Media Sponsors

Cooperation
IEICE SIG- Knowledge-based Software Engineering (SIG-KBSE)
IEICE SIG- Information-Based Induction Sciences and
Machine Learning (IBIS-ML)
Japan Deep Learning Association (JDLA)
IPSJ SIG- Software Engineering (SIG-SE)
JASPAR
NPO Association of Software Test EngineeRing (ASTER)
JSSST SIG- Foundation of Software Engineering (FOSE)
The Japanese Society for Artificial Intelligence (JSAI)

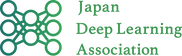
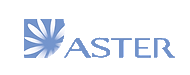
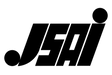
Sponsors / Supporters
Access
Hitotsubashi Hall
National Center of Sciences Building 2F,
2-1-2 Hitotsubashi,Chiyoda-ku,Tokyo 101-8439
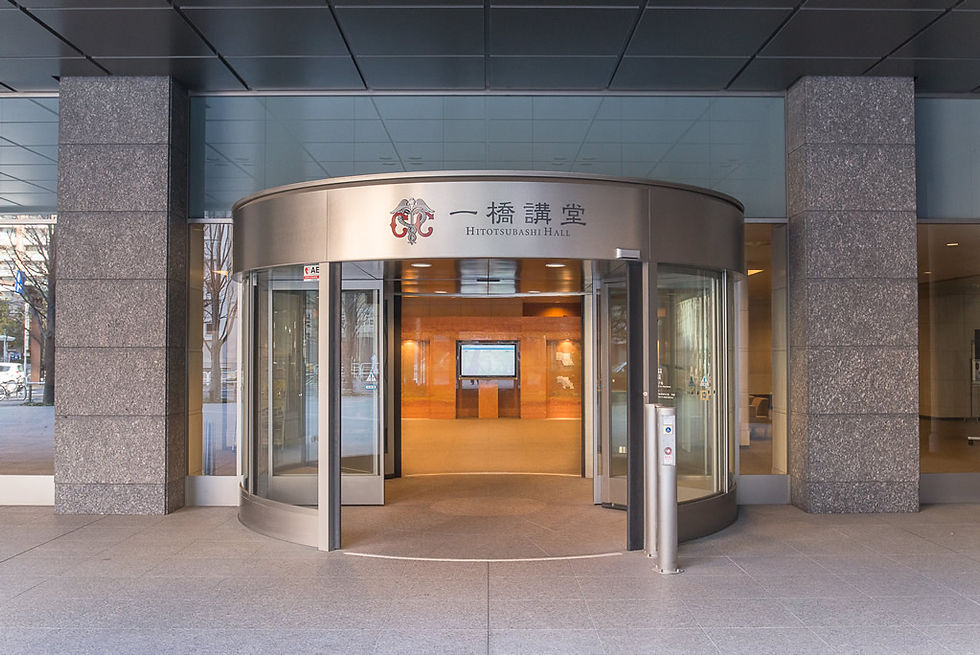
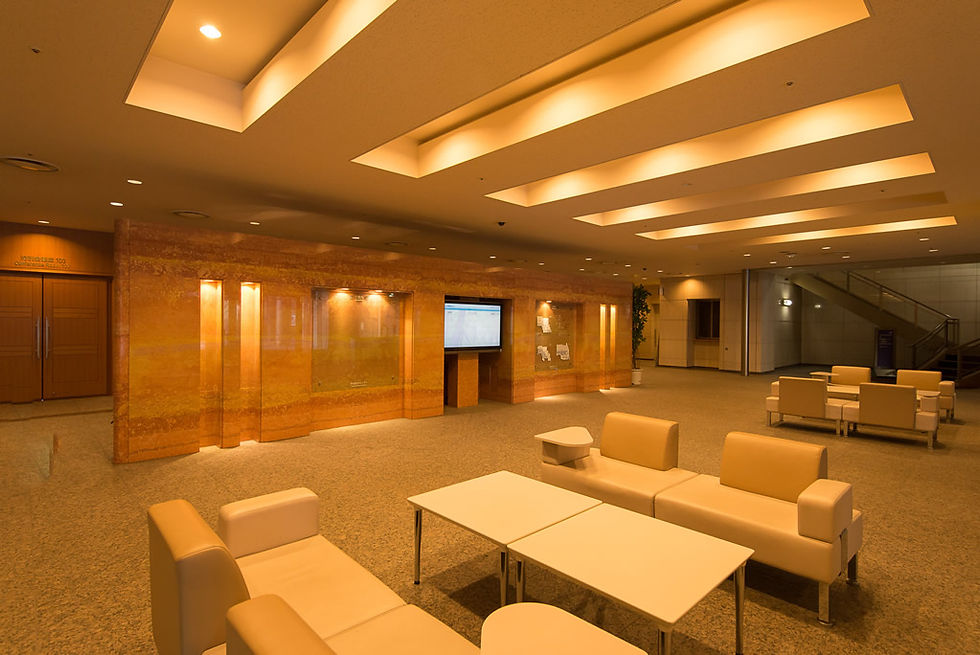
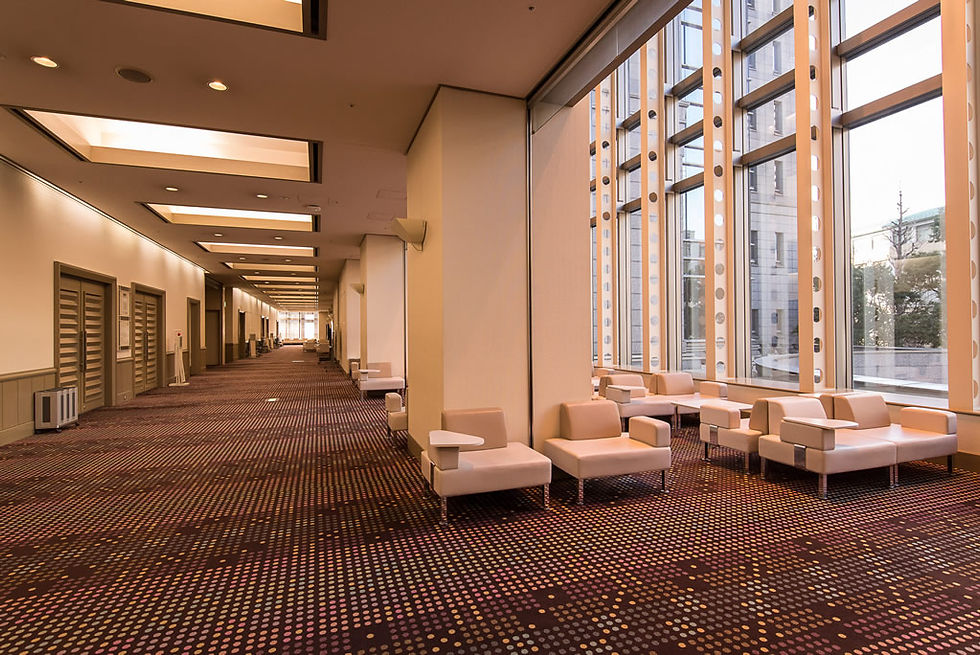
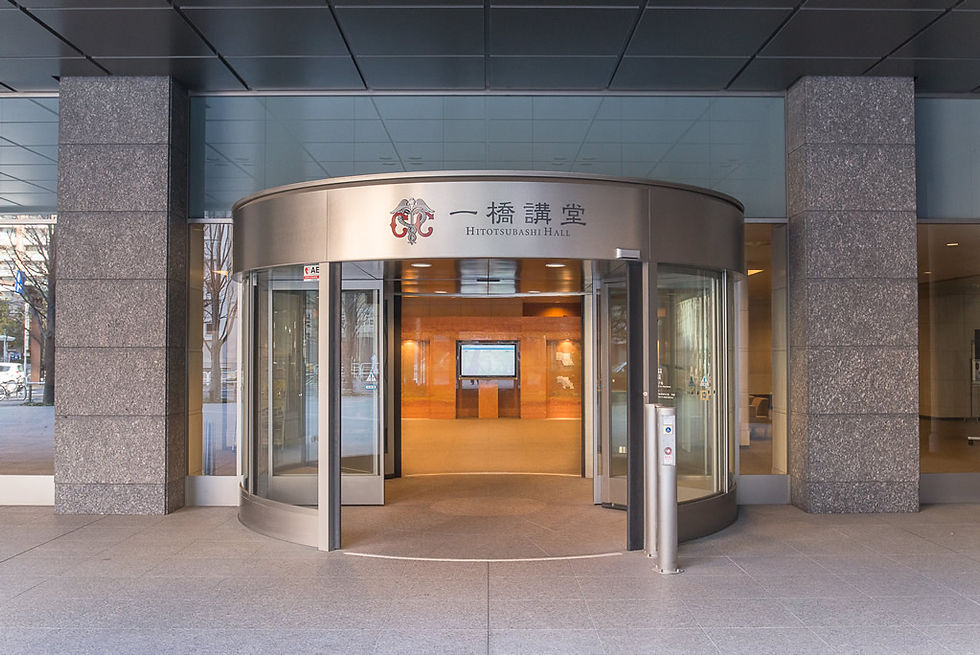
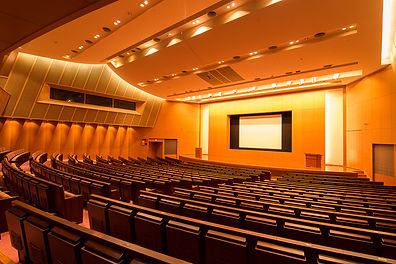